AI-predicted altcoin breakouts is a rising trend as traders use machine learning to spot opportunities, minimize risk, and optimize their crypto strategies.
The Challenge of Identifying Altcoin Breakouts
Altcoins are alternative cryptocurrencies to Bitcoin. A large number of altcoins are available in the market. While some projects gain popularity, others remain unnoticed. Figuring out which altcoin will “moon” is extremely difficult. Countless variables complicate it to find answers.
Old-school ways to analyze, like manual charting or fundamental research, consume time and usually hamper capturing the crypto markets’ complexity. With the help of machine learning, we can understand insights hidden within the data and find vacancy and breakout candidates.
Building a Machine-Learning Model for Altcoin Prediction
A good ML model to predict altcoin breakouts has to use different data sets that show different aspects of the market. The contribution of each data type to the prediction process.
1. On-Chain Data: The Backbone of Blockchain Insights
On-chain data gives a clear understanding of what’s happening on a crypto’s blockchain. Key metrics include.
- Transaction Volume: Displays user interaction and usage.
- The metric for unique wallets that entered the network is known as active addresses.
- Low concentration: Indicates that the large holder’s addresses do not have more than 5% of the total token supply.
- Burn or mint rates monitor changes in supply dynamics like burns or new issues.
For instance, sudden growth in active addresses and increasing transaction volumes can imply a growing interest in the project.
2. Analyzing the community on social media
The crypto narratives are primarily shaped by social media platforms, mainly by Reddit, Twitter and Telegram. Machine-learning algorithms can analyze.
- People have been mentioning specific altcoins a lot lately.
- Sentiment Analysis: Whether conversations are positive, negative or neutral.
- The reach and credibility of an influencer promoting a coin.
Genuine influencers often lead a positive movement to start a price rally.
3. Technical Tools for Market Analysis
- By studying the price and volume data of a stock, trend identification is done through analysis. Common indicators used in ML models include.
- The RSI (Relative Strength Index) indicates whether a market is overbought or oversold.
- Moving averages show the direction of a trend and possible reversal points.
- Bollinger Bands: Shows potential for assets to break out.
- Shows buy/sell pressure at key price levels.
Combining these indicators, ML models may recognize the patterns displayed before an impending breakout.
How Machine Learning Models Work Together
The power of AI lies in its unique capability to combine datasets to create predictions. Here’s how the process unfolds.
Step 1: Gathering and Getting Ready with Data
We gather and clean raw data from on-chain analytics platforms like Glassnode and Santiment, social listening platforms like LunarCrush, and technical charting services like TradingView. Values are imputed, and irrelevant noise is filtered out.
Step 2: Feature Engineering
Important features like transaction count growth rates, sentiment scores, and RSI divergence are extracted and normalized for the model input.
Step 3: Training the Model
Techniques for supervised learning, such as random forests or gradient boosting machines (GBMs) or neural networks, can be trained on past breakout events over historical data. The model trains to find successful breakout combinations.
Step 4: Validation and Testing
The performance of the trained model is checked on unseen data. Using metrics such as precision, recall, and F1-score ensures the model works well in different situations.
Step 5: Deployment and Monitoring
The model is in the market after the validation process. It regularly updates to respond to changing market situations.
Case Study: Predicting Dogecoin’s Surge in 2021
There was a growing adoption as the number of active addresses and transaction volume increased.
- Social Sentiment: Positive mentions soared following tweets by Elon Musk, registering all-time high values.
- Technical Patterns: Roger breakout formations above resistance continue upward momentum.
- An AI model that takes all of this into consideration would likely create a flag for DOGE as a high-probability breakout weeks before the price pumped.
Benefits and Limitations of AI-Powered Predictions
Machine learning has advantages but also challenges that come with it.
Benefits
- Scalability: Processes millions of data points faster than humans.
- Being objective = Less emotional in decisions.
- Identifies breakout signals before they become obvious to the rest of the market.
Limitations
- Bad data leads to bad models in AI/ML.
- Models may do well with historical data but can fail in reality.
- Pump-and-dump schemes can distort signals and fool algorithms.
Final thoughts: Utilize an AI for an altcoin strategy
Machine learning is a great way to predict altcoin breakouts and offers some of the best advantages. Combining data on-chain, social media sentiment, and technical indicators can help AI models uncover promising projects likely to explode during the next bull run.
Nevertheless, success hinges on thorough validation, ongoing refinement, and an understanding of the limitations of algorithmic forecasts. Investors who are willing to run with this technology could benefit because machine learning moonshots offer unique opportunities in the crypto world.
Conclusion
Altcoins can be predicted using machine-learning models. They use on-chain data as well as certain social media sentiments, like transaction volume or blockchain activity as well as price graphs like RSI. AI systems pick up on patterns that indicate a breakout on a security, allowing investors to capitalize on high-growth situations. Such models are very effective but require careful calibration and constant monitoring to prevent risks like overfitting and market manipulation. When used responsibly, AI predictions are an edge in a volatile yet lucrative altcoin market.
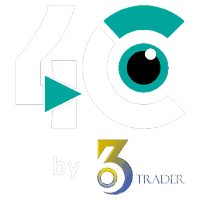